Despite being one of the most powerful computing platforms, and being free at the same time, R still struggles against other statistical software, such as SPSS and SAS, in gaining mass appeal amongst users of statistical and market intelligence software. Many have cited the absence of a user-friendly graphical user interface (GUI) for R to be partially responsible for its limited success outside the community of dedicated quants.
A new GUI for R, Deducer, is about to change R’s geeky unfriendly image. Just like R, Deducer is also free and provides the point-and-click ease of a use for R. Deducer is Java-based and therefore provides an avant-garde look and feel that rivals the user-interfaces developed by SAS and SPSS.
While Deducer is not the first or the only GUI available for R, it is indeed one of the more functional GUIs with the potential for mass appeal. An earlier issue of the journal reviewed R Commander, a popular R GUI, which was developed by Professor John Fox of McMaster University. Deducer is however different from previous attempts to add a user interface to R. First, it is aesthetically pleasing and easy to use with improved interactive dialogue boxes. Second, it is built on newer algorithms coded in R and therefore the output is formatted to meet the every day needs of the analysts. Thirdly, it offers the user interface for the famed R package for graphics, ggplot2, which is fast becoming the gold standard for analytic graphics.
Deducer has been developed by Ian Fellows, a Ph.D. student in statistics at UCLA, who relied on the functionality already built into JGR, a short for the Java GUI for R. Ian wanted Deducer to be “user friendly enough to be used by someone just starting out, yet flexible and powerful enough to increase the efficiency of expert users.” Deducer comes very close to the objectives set by Ian Fellows.
Installing Deducer is a three-step process. First, you will have to install a newer version of R[1]. Version 2.12.0 or later is recommended by the developer. The second step involves installing JGR launcher[2]. Once JGR launcher has been installed, run R and install packages JGR and Deducer. You can exit R and launch Deducer from within JGR for future use. Deducer adds additional menus to the JGR user interface for data analysis and graphics. Ian Fellows has developed another package, DeducerExtras, which provides user interfaces for additional statistical routines.
There is however a one-step installation option available for Windows only. Further details on one-step installation are listed at:
http://www.deducer.org/pmwiki/pmwiki.php?n=Main.WindowsInstallation
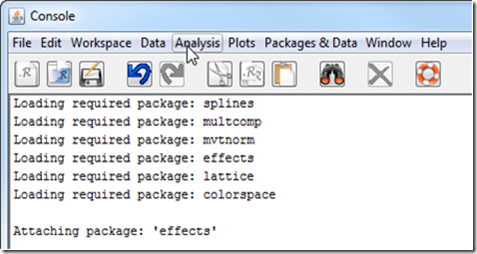
Figure 1
Users can import data from SPSS, SAS, Stata, DBF, CSV, and other formats in addition to reading data in native R formats. Once the data have been loaded into R, the user can view the data in Data Viewer, which is similar to SPSS and offer two views: data view and the variable view. Data view provides the typical tabular display of data, whereas the variable view presents the Meta data offering details about variable types, and for categorical variables information about factor levels (Figure 2). The variable view is a useful tool to quickly glance through the data to determine how each variable could be used in the analysis. If two or more data sets are loaded, the same data viewer can be deployed to view multiple data sets by selecting the required data set from a dropdown box.
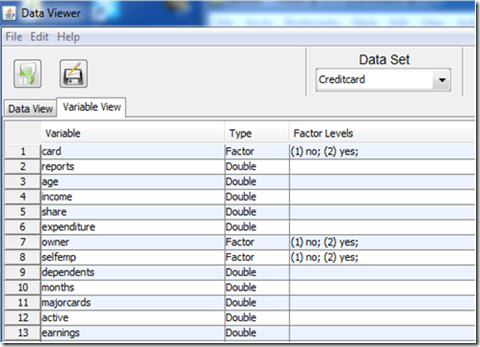
Figure 2.
The analysis menu offers the standard analysis tools varying from descriptive statistics and crosstabs to hypothesis testing. On the modelling front, Deducer offers Anova, OLS Regression, Binomial Logit models, and Generalized Linear Models. The advanced add-in package, DeducerExtras, offers further functionality for hypothesis testing and multivariate analysis.
Deducer offers highly functional dialogue boxes, which also retain in memory choices made in the last deployment. All dialogue boxes in Deducer offer a dropdown menu to select a data set for the analysis, in case two or more data sets are concurrently opened. Variables can be dragged and dropped into the dialogue boxes. Results can be organized by filtering the data as well as using one or more variables to stratify the output.
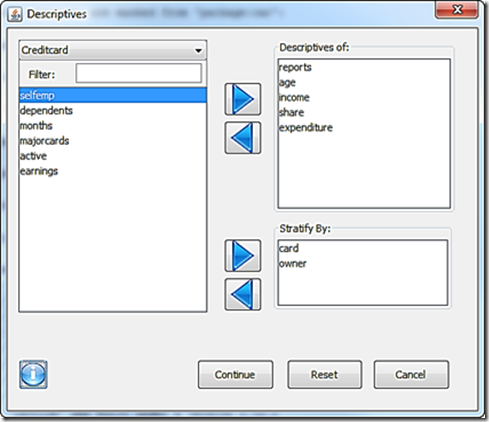
Figure 3
I illustrate Deducer’s analytic capabilities using a dataset about credit card approvals, which comes embedded in a package in R. Deducer generates output in fixed width text format. A crosstab between two categorical variables, credit card application status and home ownership broken down by a stratification variable, self-employed status, is presented below.
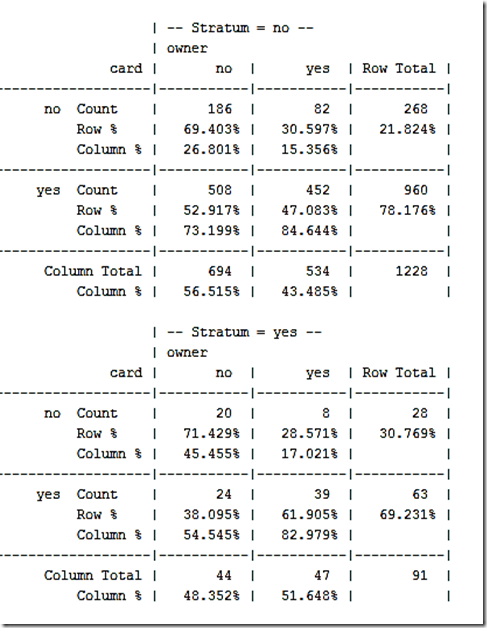
Figure 4
Deducer also generates a series of tests to determine the statistical significance of the results obtained from the cross tabulations.
Deducer’s dialogue boxes for statistical model, such as regression are very similar to those in SPSS. The dialogue box allows the user to introduce explanatory variables either as continuous or categorical variables. Furthermore, the dialogue box also offers the option to create interactions between variables by pointing and clicking. Once the model is specified, Deducer displays the output in another dialogue box so that the analyst may fine tune the model before the results are committed to the output window. This intermediate step of looking at the results on the fly and tweaking the model offers unique interactive functionality in Deducer that is missing in other commercial statistical software.
Deducer’s second most significant feature (its most important feature is it being free) is the interactive user interface for the ggplot (Grammar of Graphics) package. A whole host of graphic templates have been built in to Deducer for the user to develop analytic graphics, which are hard, if not straight out impossible, to create in other commercial statistical software. Apart from the fact that users can create graphics using the plot menu (see Figure 1), Deducer also generates supporting graphs as part of the statistical analysis. For instance, when I conducted the t-test to compare the income of those whose credit card application was successful against those whose application was rejected, Deducer offered the option to visualise the comparison as a box plot (see Figure 5), which it generated using the ggplot package without any additional input from me. This type of functionality in Deducer is of great use to market analysts who could generate supporting results and graphs with minimum input.
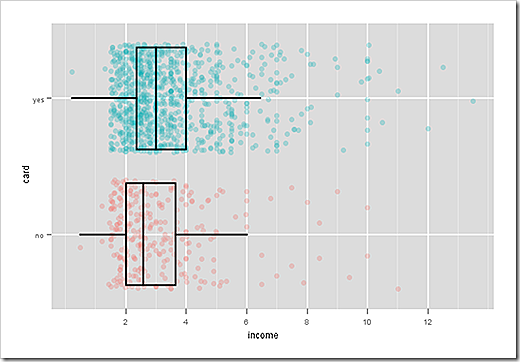
Figure 5
The numeric output can be saved as text file. Deducer offers the option to save the generated results either with or separate from the R script, which is generated by the dialogue boxes. Thus a user can save the script in a text file to re-run the analysis with modifications by simply highlighting the text and executing it with one click of a mouse.
Room for improvement
While Deducer offers dialogue boxes for the most common analytic tasks, it can broaden the scope of analytics by creating user interfaces for algorithms that have already been programmed for R. Another area of improvement is help for its dialogue boxes. Deducer offers on-line help, including text and videos. Deducer should offer off-line text-based help for instances when Internet may not be available to a user.
Another limitation in Deducer is that it does not retain the results of a statistical routine, such as a regression model, as a temporary object, which could be manipulated later. For instance, one can assign the results from a model to an object, e.g., a variable, and review and manipulate the results later by reviewing the object without executing the model again. However, Deducer removes such temporary objects by default, which may require the analyst to re-run the model from the script. Its dialogue boxes should offer the option to store results as objects for further manipulation.
I see no reason to keep Deducer and Deducer Extras separate. Currently one has to launch Deducer and DeducerExtras to get the functionality embedded in the two packages. They should be merged so that the user is not required to launch two separate packages every time.
Final Word
For businesses and individuals facing tight budgets, Deducer can be a very helpful tool that could be deployed across teams without worrying about hundreds of thousands of dollars in software licensing fees. Deducer has made R as easy to work with as other commercially available software. It is pleasing to look at and generates presentable reports and stunning analytic graphics.
[1] Download the latest version of R from http://cran.r-project.org/.
[2] Download JGR launcher from http://rforge.net/JGR/.